The Second International Workshop on Trustworthy Artificial Intelligence for Healthcare (TAI4H)
Speakers

Title: A multi-modal deep learning model based automatic Wisconsin Gait Scale scoring system for stroke patients
Abstract: Gait impairments are a common consequence of stroke and assessing gait patterns is crucial for developing personalized rehabilitation programs. The Wisconsin Gait Scale (WGS) is an effective score criterion for evaluating gait quality in stroke patients. However, the manual scoring process is labor-intensive and often subject to biases due to the varying experience levels of the clinic practitioners. Deep learning techniques offer a potential solution to this challenging difficulty. We propose STAT-Net, a novel multi-modal deep learning model inspired by biomechanical properties and comprehensive insights for automatic WGS scoring based on skeleton data collected by the Inertial Measurement Unit (IMU). We also compare the performance of different models on the task to show the superiority of our method.
Prof. Yancheng Yuan is an assistant professor in the Department of Applied Mathematics at the Hong Kong Polytechnic University. His primary research interests lie in the theoretical and applied aspects of continuous optimization and machine learning. He has published papers in prestigious journals such as the "SIAM Journal on Optimization," "Journal of Machine Learning Research," and "IEEE Transactions on Neural Networks and Learning Systems", as well as presented at top academic conferences in the field of machine learning such as ICML, NeurIPS, SIGIR, and WWW. He has received the Best Paper Award Finalist (WWW 2021).
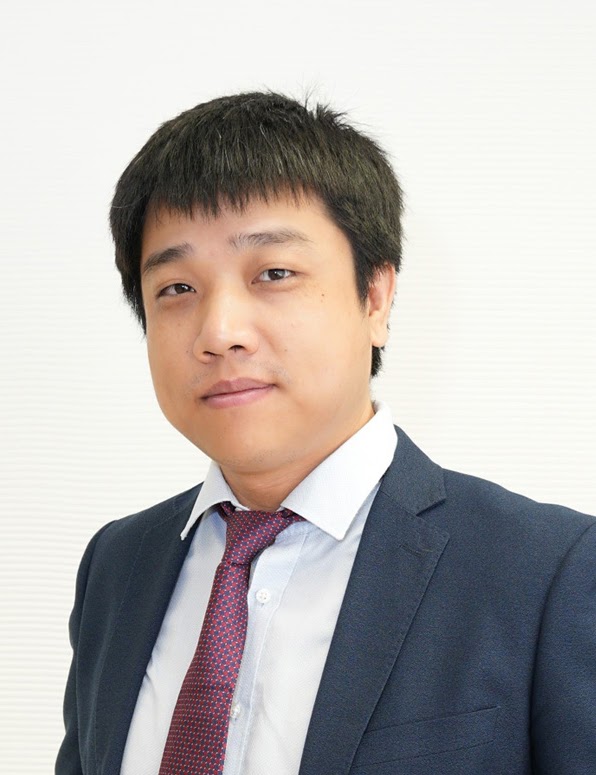
Title: Transcriptomic, histopathological and radiological deep learning models for cancer subtype classification
Abstract: It is now clear that major human cancers are heterogeneous diseases, which hamper the selection of patients for optimized clinical management and treatment. To dissect the complex cancer heterogeneity, molecular subtyping has been widely adopted as an effective strategy in the last couple of decades. Despite the insights into cancer biology, the conventional cancer subtyping studies were mostly based on conventional machine learning methods applied to transcriptomics data, which lack cross-platform robustness and are not suitable for clinical translation. In this talk, I will first introduce a deep learning framework based on transcriptomic profiles, which is platform independent, robust to missing data, and can be used for single sample prediction. To facilitate clinical implementation, I will next introduce our recent works on deep learning models for cancer subtyping based on histopathological images and radiological scans. These medical imaging data is widely available in the clinic, hence more suitable for the translation of cancer subtyping into real clinical practice to advance precision oncology.
Prof. Xin Wang obtained his PhD in 2014 from the University of Cambridge Department of Oncology and Cancer Research UK Cambridge Institute. From 2013 to 2015, Prof. Wang did his postdoctoral research at the Department of Biomedical Informatics, Harvard Medical School. He is currently an Associate Professor at the Department of Surgery, Chinese University of Hong Kong, directing the Division of Biomedical Informatics and MPhil-PhD Programme in Translational Genomics. He is currently also leading the Laboratory of Translational Bioinformatics at LI Ka Shing Institute of Health Sciences as a Principal Investigator.
Prof. Wang’s major research field is cancer genomics and bioinformatics. Since 2012, he has been focusing on mechanistic and translational research in major human cancers by developing novel methodologies integrating bioinformatics, systems biology, machine learning and artificial intelligence. In collaboration with molecular biologists and experimental oncologists, he is especially fascinated by the research into the mechanisms underlying cancer development and metastasis. Together with clinicians, his group has also been dedicating to multi-center studies about molecular and image-based biomarkers for cancer early detection, diagnosis, prognosis and subtyping. Prof. Wang published > 90 papers in well-known journals such as Nature Medicine, Gastroenterology, Hepatology, Annals of Surgery, Science Advances, and Nature Communications, with > 10,000 citations. His work is currently supported by significant research funds by Research Grants Council of Hong Kong, Shenzhen City and Guangdong Province, as well as National Natural Science Foundation of China.

Title: A Generalized Label-efficient Framework for Medical Image Segmentation and a new Benchmark for Colorectal Cancer Segmentation
Abstract: Medical image segmentation plays a crucial role in clinical settings, augmenting the accuracy of diagnosis, treatment planning, and disease surveillance. Here we present a generalized weakly supervised framework for medical image segmentation, termed WeakMed, to alleviate the reliance on costly annotations while being tractable to various scenarios. Specifically, the proposed WeakMed method creatively utilizes the mask-to-box transformation to overcome rectangular shape biases inherent in bounding box labels by leveraging economical bounding box annotations, thus largely streamlining the annotation of medical data. Besides, WeakMed is a versatile model, enabling adaptation to a variety of segmentation tasks and bringing no computation costs to model inference. Through rigorous evaluation across 9 segmentation tasks, 10 diverse medical datasets, and 6 distinct imaging modalities, WeakMed demonstrates superior accuracy and robustness when compared to its fully/weakly supervised counterparts. By alleviating the data annotation burden associated with a broad spectrum of segmentation tasks, WeakMed has the potential to advance diagnostic accuracy and facilitate the development of personalized treatment strategies. Besides, we collected and annotated the first benchmark dataset that covers diverse ERUS scenarios, i.e. colorectal cancer segmentation, detection, and infiltration depth staging. Our ERUS-10K dataset comprises 77 videos and 10,000 high-resolution annotated frames.
Dr. Zhen Li is currently an assistant professor at the School of Science and Engineering (SSE) of The Chinese University of Hong Kong (Shenzhen)/Future Intelligent Network Research Institute (FNii)-Shenzhen. Dr. Zhen Li was selected for the Wu Wenjun Outstanding Youth of 2023, 2021-2023 Seventh China Association for Science and Technology Young Talent Support Project. His research interests include interdisciplinary research in artificial intelligence, 3D vision, computer vision, and deep learning-assisted medical big data analysis. He has published over 60 papers in top conferences and journals, such as top journals Cell Systems, Nature Communications, IEEE TPAMI, IEEE TNNLS, IEEE TMI, PLOS CB, Bioinformatics, JCIM etc., and top conferences CVPR, ICCV, ECCV, NeurIPS, ICLR, MM, AAAI, IJCAI, ACL, ECAI, MICCAI, RECOMB, etc.

Title: TBD
Abstract: TBD
Prof. Yifan Peng is an assistant professor in the division of Health Sciences at Weill Cornell Medicine. His main research interests include BioNLP and medical image analysis, such as named entity recognition, information extraction, and disease diagnosis and prognosis. Before joining Cornell Medicine, He was a research fellow at the National Center for Biotechnology Information (NCBI), National Library of Medicine (NLM), National Institutes of Health (NIH). He obtained his Ph.D. degree from the University of Delaware. During his doctoral training, he investigated applications of machine learning in biomedical relation extraction, with a focus on deep analysis of the linguistic structures of biomedical texts. He is the first awardee at the NCBI to receive the NIH K99/R00 grant, which supports his work on using NLP and ML to extract radiology specific domain knowledge and build an automated reporting system.